In an innovative leap, researchers are turning to the natural complexities of the human brain, attempting to mimic its efficiency using a novel technology – nanomagnets.
Leading this groundbreaking research is Benjamin Jungfleisch, an associate professor at the University of Delaware, who is working on using these nanomagnets to pave the way for more energy-efficient artificial intelligence (AI) systems.
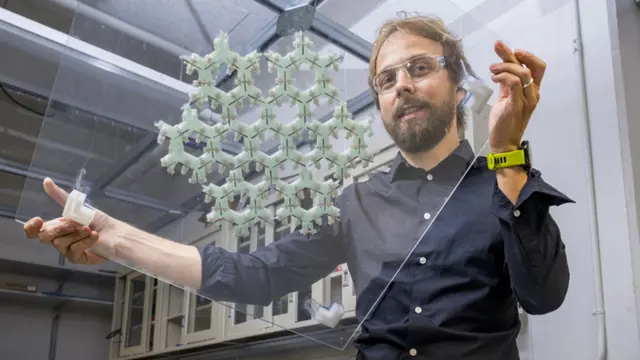
The motivation behind this research is clear: with the rise of AI technologies and tools like ChatGPT, the demand for computing power is skyrocketing, resulting in increased energy consumption.
Jungfleisch aims to address this concern through an approach inspired by the brain’s ability to process and retain information while consuming only a fraction of the energy that modern AI systems require.
Central to this approach is a field known as magnon spintronics.
Here, magnons—the fundamental quantum excitations responsible for creating magnetic waves—serve as the information carriers, much like neurons in the brain.
When placed in networks, these tiny nanomagnets act similarly to neurons and their synapses, which are responsible for signal transmission pathways.
“These arrays of interacting nanomagnets are essentially just tiny bar magnets like the ones you have on your fridge,” Jungfleisch explains.
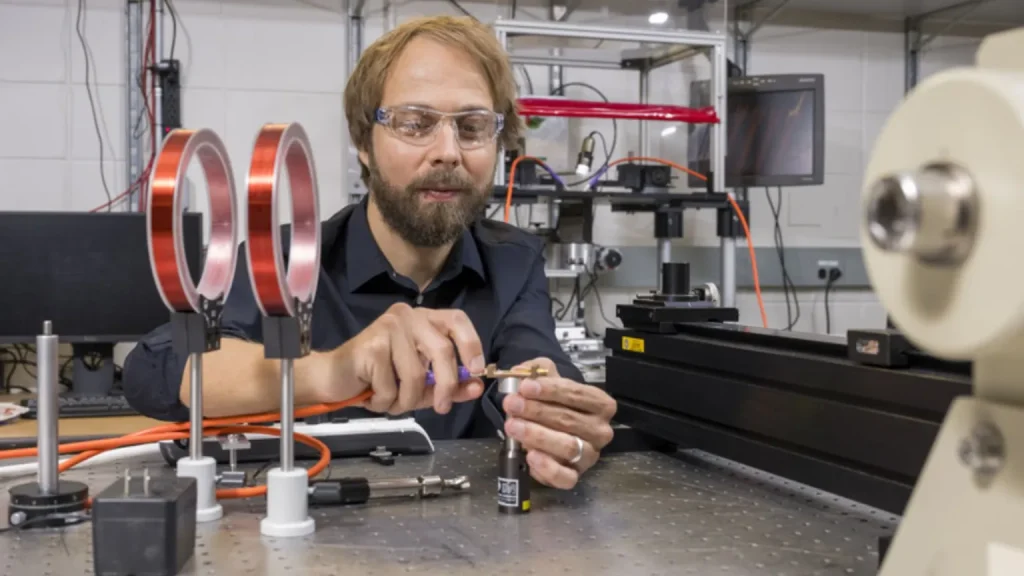
“At the nanoscale, you can pattern them with the latest lithography techniques, forming lattices that interact much like neurons.”
This allows the nanomagnets to both store and process information in a unified manner, unlike traditional computing, which separates memory and processing units.
This intriguing resemblance to neural operations offers significant advantages.
By using magnetic excitations instead of electrons to handle data, these nanomagnetic systems offer a unique dual function – simultaneously acting as memory storage and processors.
This leads to a reduction in energy usage without sacrificing performance.
Another promising feature of this technology is its ability to store a historical record of states and be trained akin to a human brain.
Jungfleisch and his team are currently working on optimizing these training cycles, which presently take a few hours but could become significantly quicker.
Moreover, the team has developed a three-dimensional nanomagnetic structure that can be easily fabricated using established techniques, providing more states for data storage within a smaller physical footprint.
“This provides more space available for storing information and offers reconfigurable memory and synaptic behavior that could lead to more adaptable computing systems,” noted Jungfleisch.
The implications of this research extend beyond enhanced AI systems.
By developing these energy-efficient computing strategies, Jungfleisch and his team are contributing to the sustainable future of technology, where energy demands do not compromise environmental health.
This research opens exciting new pathways in the quest for high-performance, energy-efficient technology, potentially redefining AI’s role in a world increasingly reliant on sustainable initiatives.